This page was generated from nldas.ipynb.
Interactive online version:
NLDAS2 Forcing#
[1]:
from pathlib import Path
import pynldas2 as nldas
from pygeohydro import WBD
The NLDAS2 database provides forcing data at 1/8th-degree grid spacing and range from 01 Jan 1979 to present. Let’s take a look at NLDAS2 grid mask that includes land, water, soil, and vegetation masks:
[2]:
grid = nldas.get_grid_mask()
grid
[2]:
<xarray.Dataset> Size: 2MB Dimensions: (lon: 464, lat: 224, time: 1, bnds: 2) Coordinates: * lon (lon) float32 2kB -124.9 -124.8 -124.7 ... -67.31 -67.19 -67.06 * lat (lat) float32 896B 25.06 25.19 25.31 ... 52.69 52.81 52.94 * time (time) datetime64[ns] 8B 2000-01-01 spatial_ref int64 8B 0 Dimensions without coordinates: bnds Data variables: time_bnds (time, bnds) datetime64[ns] 16B ... NLDAS_mask (time, lat, lon) float32 416kB ... CONUS_mask (time, lat, lon) float32 416kB ... NLDAS_veg (time, lat, lon) float32 416kB ... NLDAS_soil (time, lat, lon) float32 416kB ... Attributes: (12/13) missing_value: -9999.0 time_definition: constant title: NLDAS masks and predominant vegetation/soil institution: NASA GSFC history: created on date: Fri Mar 8 15:58:50 2019 references: Mitchell_etal_JGR_2004; Xia_etal_JGR_2012 ... ... website: https://ldas.gsfc.nasa.gov/nldas/ MAP_PROJECTION: EQUIDISTANT CYLINDRICAL SOUTH_WEST_CORNER_LAT: 25.0625 SOUTH_WEST_CORNER_LON: -124.9375 DX: 0.125 DY: 0.125
For example, let’s plot the vegetation mask.
[3]:
ax = grid.NLDAS_veg.plot()
ax.figure.savefig(Path("_static", "nldas_grid.png"), facecolor="w", bbox_inches="tight")
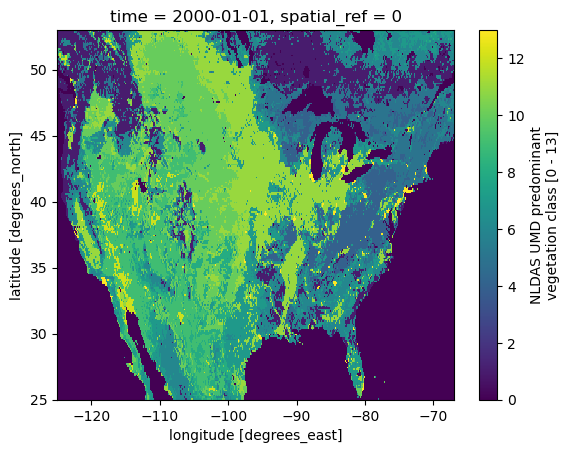
Next, we use PyGeoHydro to get the geometry of a HUC8 with ID of 1306003:
[4]:
huc8 = WBD("huc8")
geometry = huc8.byids("huc8", "13060003").geometry.iloc[0]
PyNLDAS2 allows us to get the data for a list of coordinates using pynldas2.get_bycoords
or for a region as gridded data using pynldas2.get_bygeom
. Here, we use the latter. Note that if we don’t pass any variables, all variables will be downloaded. Additionally, we can pass snow=True
to separate snow portion of precipitation using temperature. PyNLDAS2 provides access to NLDAS2 data from two sources: grib
and netcdf
. They are mostly
similar so if for some reason one of the sources is not temporarly available, you can use the other one. The default source is grib
.
[5]:
clm = nldas.get_bygeom(geometry, "2010-01-08", "2010-01-08", 4326, variables="prcp", snow=True)
[6]:
ax = clm.snow.sel(time=slice("2010-01-08T05:00:00", "2010-01-08T010:00:00")).plot(
col="time", col_wrap=3
)
ax.fig.savefig(Path("_static", "nldas_snow.png"), facecolor="w", bbox_inches="tight")
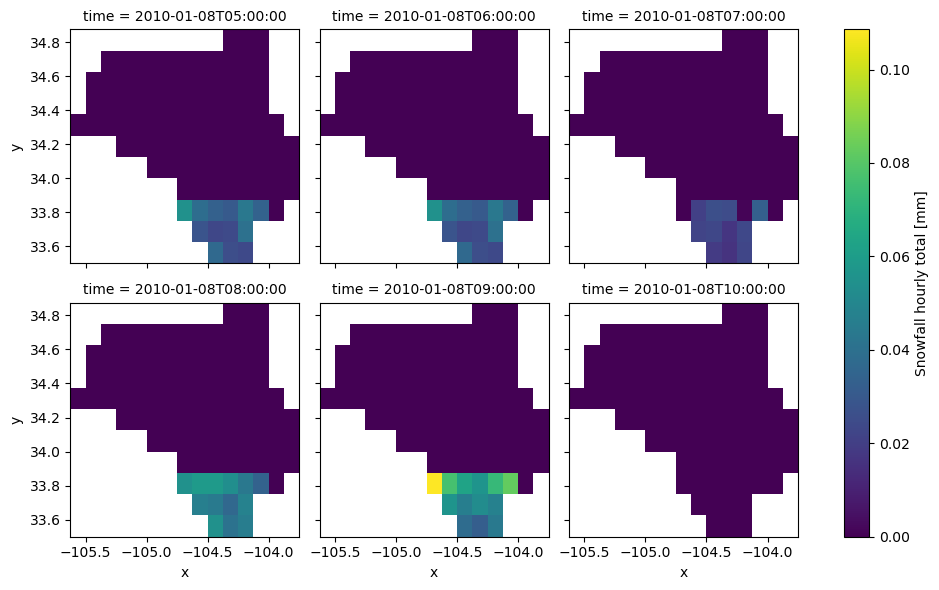